Research
We investigate the computational principles and mechanisms that underlie neuromechanical control and motor learning. Our work uses a blend of experimental, theoretical and computational approaches. We focus primarily on the human arm as a system that demonstrates many of the features that make motor control a difficult problem (Franklin and Wolpert, 2011, Neuron). We use robotic interfaces and virtual reality systems in order to control precisely the sensory inputs to the subjects.
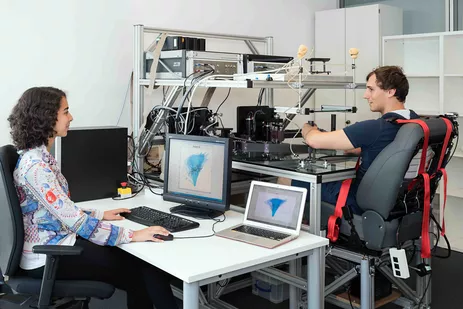
Our research focus is primarily within five different topics:
Motor memory formation: How do humans learn models of the external world and use these to adapt our movements to new experiences? We have shown that there is a critical time before and after movements in which the active motor memory can be accessed and modulated (Howard et al., 2012, J Neurosci; Howard et al., 2015, Curr Biol) and that motor planning is more critical than execution (Sheahan et al., 2016, Neuron).
Motor memory representation: In what manner are these motor memories represented within the brain? We have shown that motor memories are represented in a mixed co-ordinate system that includes Cartesian, joint and object-based coordinates (Berniker et al., 2013, J Neurophysiol; Franklin et al, 2016, eNeuro).
Feedback modulation: We have developed novel techniques to measure the visuomotor feedback gains and shown how they are modulated according to the environment (Franklin and Wolpert, 2008, J Neurosci; Franklin et al., 2012, J Neurophysiol)
Visuomotor Control: We use visuomotor feedback gains in order to probe the computations underlying visual control of reaching movements (Dimitriou et al., 2013, J Neurosci; Reichenbach et al., 2014, Curr Biol; Franklin et al., 2016, J Neurosci).
Neuromechanics: We have shown that the nervous system can modulate the limb stiffness independently of endpoint force, tuning it directionally to instability in the environment (Burdet et al., 2001, Nature; Franklin et al., 2007, J Neurosci; Selen et al., 2009, J Neurosci).